
The Good, The Bad, and The Rock Fights: Sonny Dykes Edition
Or, how Sonny Dykes learned to stop The Bad and love The Rockfights
Earlier this season we debuted a new weekly feature where we use PFF grades from the Bears’ most recent game to classify that performance into one of three types of games: Good (offense and defense perform well), Bad (offense and defense struggle), and Rockfights (offense struggles while defense excels). Eventually we developed a fourth category, Pillowfights, in which the offense performs well while the defense underperforms. While we have so far focused on the Justin Wilcox era at Cal, I wondered in our initial piece how the Sonny Dykes era would break down in a similar analysis. And now that Dykes is days away from leading TCU into the playoffs, now seems like a fine time to look back on his teams at Cal and how his 12-win TCU team compares. Over the Sonny Dykes era at Cal many of us remember a bunch of blowout losses (the entire 2013 season), scoreboard-breaking games (Goff-era shootouts against Colorado, Wazzu, and Arizona), and occasional blowout wins (Oregon State). Will we indeed see a breakdown along those lines? Before we dive into the results, let’s review what is tracked in the PFF data.
PFF Data Overview
After each game Pro Football Focus provides grades across twelve distinct categories spanning offense and defense. Here is the full list of categories:
Overall performance
Offense
Passing
Pass protection
Receiving
Running
Run blocking
Defense
Run defense
Tackling
Pass rush
Pass coverage
Grades in each category range from 0 to 100. Anything below 30 is eye-bleachingly bad (think of the Cal passing attack in the Cheez It Bowl), 30-50 is bad, 50-60 is mediocre, 60-70 is decent, 70-80 is pretty good, 80-90 is excellent, and 90+ is spectacular (think Jaydn Ott’s breakout game against Arizona this season). Below I have plotted boxplots capturing the range of grades for Sonny Dykes’ 2014-16 Cal teams and his 2022 TCU team. Mercifully, PFF did not grade the 2013 Cal games so they are excluded from this analysis.
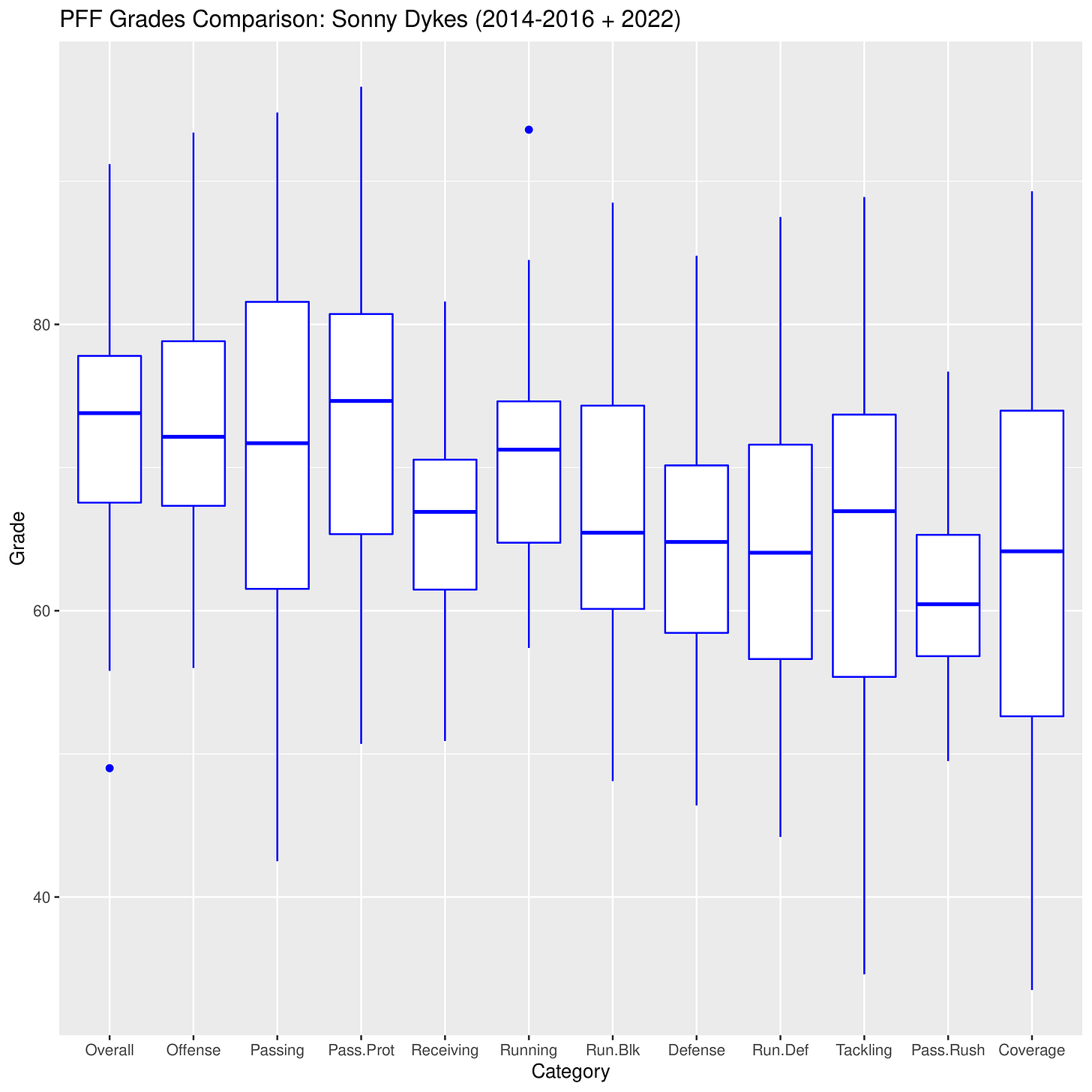
If you’ve been following my The Good, The Bad, and The Rockfights series or the Rating the Bears series this season, you’ve probably read the description of a boxplot dozens of times. But for the uninitiated, here’s a quick description of what the above plot tells us: the horizontal blue line in the middle of the box represents the midpoint of the data while the surrounding box captures all data between the 25th and 75th percentiles. The vertical lines above and below the box respectively capture the upper end and lower ends of the data, and any solitary blue dots represent extreme outliers.
As much as we associate Sonny Dykes with a stellar passing attack, the category with the highest typical grade is pass protection. Despite throwing the ball 40-50 times per game compared to 20-35 times per game under Wilcox, Dykes’ Cal offenses typically surrendered about 2-2.2 sacks per game, well below the typical Wilcox team. Passing and offense are nearly tied for second-highest typical grade. Complementing the pass-happy attack, Dykes’ RBs rate quite well. Interestingly, his receivers have not been graded nearly as well as the rest of the offense. And despite the excellent grades for running, the run blocking consistently failed to achieve the same stellar grades as the rest of the offense. Those two aspects, receiving and run blocking, were the only two weaknesses on offense.
Speaking of weaknesses, defense was a consistent and often insurmountable weakness for Dykes’ teams at Cal. Pass rush is consistently the worst aspect of Dykes’ defenses. And as Cal fans have lamented in recent years, a weak pass rush will make the linebackers and DBs look much worse. After that, most other categories were consistently rated quite poorly. Interestingly, the big boxes and bars for the tackling and coverage categories indicate that Dykes’ teams’ performances were all over the place in those categories. Sometimes they’d perform well and sometimes defenders tackled like their opponents were coated in teflon.
For years Cal fans have wondered what could have happened if a defense as solid at Wilcox’s could have been matched with an offense as prolific as Dykes’. At least, we had wondered about that until his 2022 TCU team managed to match a typical Sonny Dykes offense with a defense about on par with Wilcox’s teams (excluding the elite 2018 unit, however).
Building Clusters of Prototypical Sonny Dykes Performances
We have 50 games of data across the 2014-16 Cal teams and the 2022 TCU team. Spread across 12 categories, that’s 600 data points. There are too many data points to plot and try to identify specific clusters of games using the eyeball test (or the inter-ocular percussion test, to use the official statistical terminology). Instead, we turn to machine learning to find clusters within the data. We use a k-means clustering algorithm to identify clusters of similar games in the data. Each cluster represents a similar subset of games. If you want to learn more about the k-means clustering algorithm and how it was utilized here, I have provided plenty more details in the appendix at the end of this piece. Otherwise, keep reading for the results.
The clustering algorithm identified four clusters of games in the data. That is, there are four prototypical types of games under Sonny Dykes. Amusingly, they roughly map onto the same four clusters we’ve seen under Justin Wilcox: the good (solid grades all around), the bad (everything is awful), the rockfights (struggling offense, good defense), and the pillowfights (good offense, bad defense). The color-coded plot below shows the relative grades for each of the four game types (note: the data points were rescaled before being input into the clustering algorithm, so they’re no longer on that 0-100 scale).
As the name suggests, The Good is characterized by solid performances across most of the categories. Passing, receiving, pass rush, and pass coverage are particularly strong in Dykes’ Good games. Meanwhile, The Bad tends to be mostly awful, although pass blocking and running still fare reasonably well. Rockfights tend to feature strong performances from the defense, particularly the run defense and tackling, while the passing game and offensive line struggle. Pillowfights feature strong scores across all offensive categories while nearly all defensive categories (except pass rush) struggle.
Although we find the same set of categories across both Dykes and Wilcox, there are some slight differences across the two coaches’ categories: The Good under Wilcox has much better pass blocking while Dykes’ Good games have better tackling and pass rush; Dykes’ games under The Bad still manage decent running grades while Wilcox’s The Bad games tend to have better defense; and Wilcox’s rockfights are anchored by lockdown pass defense while Dykes’ rockfights are anchored by solid run defense. So while we have the same categories for both coaches, they have slightly different flavors.
The plot below visualizes the four types of Sonny Dykes games in four color-coded clusters. Blue represents The Good (interestingly, only one TCU game is present despite their 12-1 record this year), red represents The Bad (unsurprisingly, none of the TCU games fell in this category), gold represents The Rockfights (more than half of these are from his 2022 TCU season), and green represents The Pillowfights (9 out of 11 are from his Cal teams).
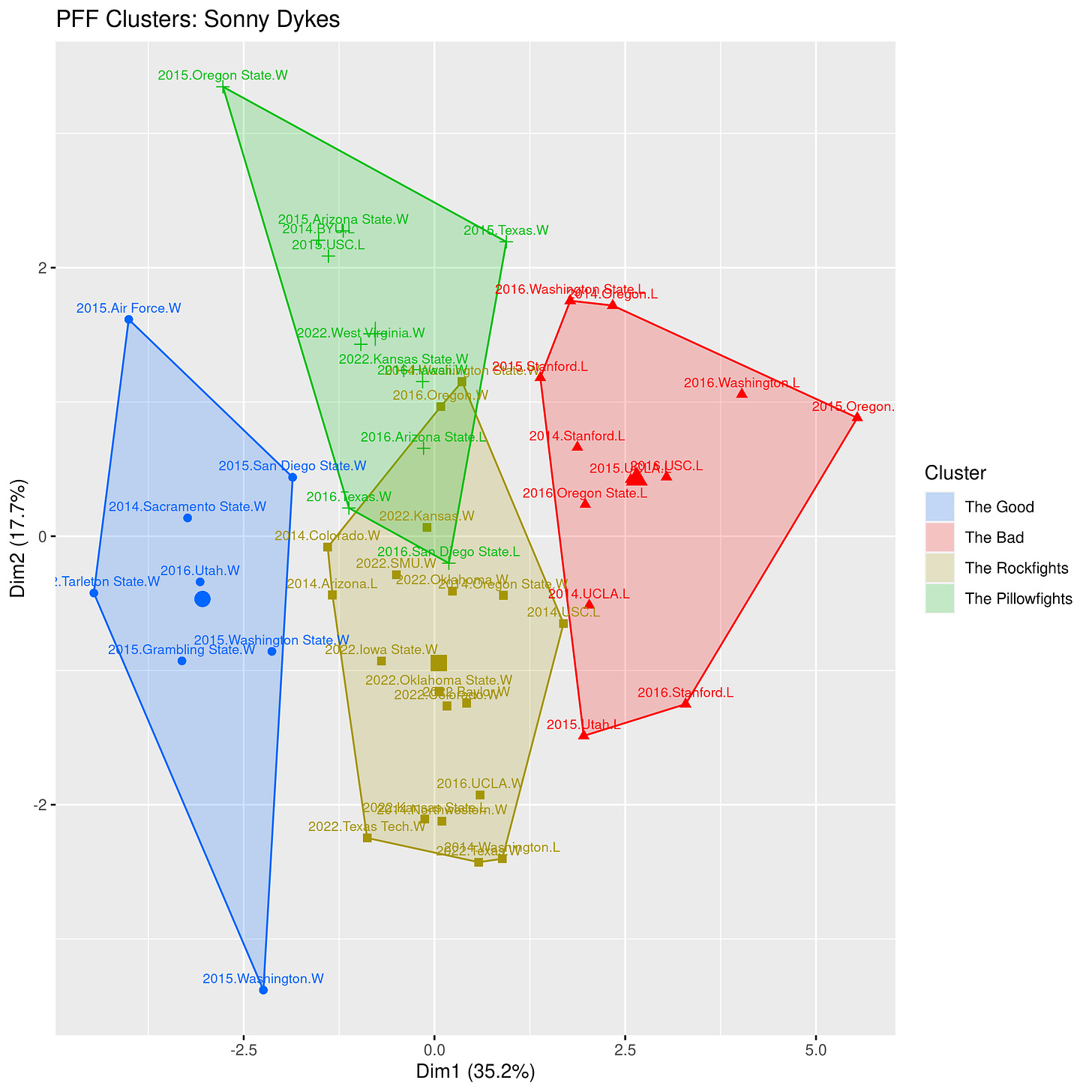
Games near each other in the plot are more similar while games farther apart are more different. The center of each cluster is marked by a larger-than-usual icon, and games near that midpoint are typical for that cluster. The most typical game of The Good cluster was the 2016 Utah game, a very atypical game for Sonny Dykes’ Cal teams. Behind Davis Webb’s 4 TDs and 306 passing yards, the Bears upset undefeated Utah thanks to a stellar defensive performance culminating in a game-sealing goal line stand. On the other end of the spectrum, the most typical game of The Bad is the 2015 loss to UCLA when the Bear Raid put up 3 TDs on an abysmal 4.8 yards per play while the defense let freshman QB Josh Rosen pass for 399 yards and 3 TDs while the UCLA ground game piled up 174 yards and another TD.
The Rockfights are full of TCU games and the most typical rockfights are the 2022 wins over Oklahoma State and Baylor, both games requiring late rallies from the Horned Frogs to preserve their then-undefeated season. Both games featured struggles from the TCU passing game (at least, relative to its stratospheric standards) while the run defense and pass coverage excelled—yep, sounds like a rockfight. Meanwhile the most typical games in The Pillowfights were TCU’s wins over West Virginia and Kansas State, another pair of games many Cal fans probably missed. However, the most typical Cal Pillowfight was the Down Under Bowl against Hawaii in the 2016 season opener, a 630-yard explosion on offense while the run defense gave up a foreboding 248 rush yards to a Hawaii team that would go on to win a whopping 3 games.
Breaking Down Clusters Year-by-Year
Year-by-year, we can see the evolution of Sonny Dykes teams over time (again, the 2013 season is mercifully ungraded by PFF). In 2014 the Bears won their lone Good game (Sacramento State), lost all three Bad games, split their 7 rockfights (4-3), and lost their lone pillowfight. In 2015 the Sonny Dykes had a career high 5 Goods (5-0, naturally), although they balanced that with 4 Bads (0-4, of course). Despite putting together his best defense at Cal in 2015 (although it still surrendered 31 points per game and an awful 6.1 yards per play), Dykes’ team did not field a single Rockfight in 2015. But that team put up 4 pillowfights and won 3 of them. In 2016 the Bears had a mere one Good (1-0) and a horrific 5 Bads (0-5); they won both Rockfights (2-0) but fared poorly in their 4 Pillowfights (1-3). Dykes’ Cal teams played Bad games almost twice as often as Good ones, but they fared reasonably well in Rockfights (6-3) and nearly split their Pillowfights (4-5).
Dykes’ 2022 TCU team had a big change from his Cal teams. In 2022 his team didn’t have a single Bad game, although they only had one Good (1-0). His team seemed to be involved in Rockfights nearly every week and they went an impressive 8-1 in those matchups. They also faced two Pillowfights and won both. So what changed from his time with the Sturdy Golden Bears to his time with the Horned Hypnotoads? The biggest difference is the elimination of The Bads, a type of game that plagued his Bears. Additionally, his teams embraced the Rockfights and fared even better in those games than his Cal teams did. How many of us would have expected that outcome after Dykes departed Berkeley in 2016? And now, his team is playoff-bound.
Appendix (shamelessly copy+pasted from our original piece on PFF clusters)
Our machine learning algorithm of choice for this application is the k-means clustering algorithm. The goal of the k-means clustering algorithm is to identify sets of clusters where data points in these clusters are much more similar to each other than they are to members of other clusters. Using a user-specificied number of clusters (the k in k-means), the algorithm starts by creating k subgroups and randomly assigning the starting positions of those clusters. It then begins an iterative process to determine cluster membership. Here’s how the iterative process works:
Starting with the first, randomly generated set of clusters, the algorithm uses all our categories (pass offense, pass blocking, run offense, run blocking, etc.) to calculate the central position in each cluster. Each data point will then be assigned to whichever cluster has the closest center. Ideally, cluster members will have values close to their cluster center and large differences to the centers of other clusters. Now that the first round of cluster membership has been assigned, the algorithm calculates the new center points in each of the clusters, and assigns data points to the closest cluster center (some data points will change clusters during this step). Once the new cluster assignments are determined, the process repeats again: calculate the centers and assign data points to their closest centers. It repeats this over and over until data points stop changing cluster membership.
Through this iterative process the algorithm aims to minimize squared euclidean distances within groups by achieving the lowest total within-cluster sum of squares (in less technical terms, the total deviation of everyone from the cluster center) and maximize squared euclidean distances across groups (maximizing deviations across clusters). It uses this iterative process to churn through various cluster memberships until the best possible fit is achieved. Ideally, we will end up with a coherent set of distinct clusters. Cluster membership can be shaped by the randomly generated starting point, so we randomly generated 25 different starting points and the algorithm chose the best one after its iterative, cluster-validating process.
One of the primary challenges here is identifying the total number of clusters. The total number of clusters in a k-means ranges from 1 (entirely uninteresting, as everyone is in the same cluster) to the total number of data points (each data point would be in its own cluster--again, profoundly uninteresting). One strategy to identify the ideal number of clusters is to run the k-means clustering algorithm on a range of values (2 clusters, 3 clusters, 4 clusters, and so on) and calculate the within-cluster sum of squares for each clustering solution. The within-cluster sum of squares should decrease consistently as more clusters are introduced, but the rate of improvement will eventually start decreasing (for those familiar with calculus, it's like taking the second derivative of a function and identifying the point at which that second derivative transitions from negative to positive). Similarly, one could look at the amount of variance explained across different clustering solutions (1, 2, 3, etc.) and identify the point at which the rate of improvement markedly slows down. Identifying the optimal number of clusters can be a subjective process, and in some cases different statistics can suggest different number of clusters. Ideally, a variety of statistics will point to the same number of clusters. But that's not always the case. There are a number of different approaches for identifying the ideal number of clusters and I encourage anyone interested in reading further about it to peruse this wikipedia page for some example approaches (https://en.wikipedia.org/wiki/Determining_the_number_of_clusters_in_a_data_set).
Why did I read this. I was having such a nice post-Christmas week.
It's hard to escape the conclusion that was also my sense at the time that while Sonny ball, the Cal version, was fundamentally flawed, the biggest issue was wild inconsistencies, which are often in turn a product of a lack of depth and breadth of talent that set up for wild swings and disasters whenever things didn't go better than could be expected.
While the locations of the problems are different in the Wilcox era, the problem persists, which suggests problems that are bigger and outside the control of Sonny or Justin that prevents assembling or maintaining an adequate staff or roster.
It would take some considerable research to confirm a similar situation exists that accounts for basketball and baseball mediocrity, but it seems entirely likely that's the case, and accounts for why what looks like aggregate talent that bounces between the middle of the Mt West and the middle of the Big West levels produces results that match.